Linear Independence Calculator
Welcome to the linear independence calculator, where we'll learn how to check if you're dealing with linearly independent vectors or not.
In essence, the world around us is a vector space and sometimes it is useful to limit ourselves to a smaller section of it. For example, a sphere is a 3-dimensional shape, but a circle exists in just two dimensions, so why bother with calculations in three?
Linear dependence allows us to do just that - work in a smaller space, the so-called span of the vectors in question. But don't you worry if you've found all these fancy words fuzzy so far. In a second, we'll slowly go through all of this together.
So grab your morning/evening snack for the road, and let's get going!
What is a vector?
When you ask someone, "What is a vector?" quite often, you'll get the answer "an arrow." After all, we usually denote them with an arrow over a small letter:
Well, let's just say that this answer will not score you 100 on a test. Formally, a vector is an element of vector space. End of definition. Easy enough. We can finish studying. Everything is clear now.
But what is a vector space, then? Again, the mathematical definition leaves a lot to be desired: it's a set of elements with some operations (addition and multiplication by scalar), which must have several specific properties. So, why don't we just leave the formalism and look at some real examples?
The Cartesian space is an example of a vector space. This means that the numerical line, the plane, and the 3-dimensional space we live in are all vector spaces. Their elements are, respectively, numbers, pairs of numbers, and triples of numbers, which, in each case, describe the location of a point (an element of the space). For instance, the number or point are elements of (different!) vector spaces. Often, when drawing the forces that act on an object, like velocity or gravitational pull, we use straight arrows to describe their direction and value, and that's where the "arrow definition" comes from.
What is quite important is that we have well-defined operations on the vectors mentioned above. There are some slightly more sophisticated ones like the dot product and the cross product (if you need to learn the difference between them, visit the cross product calculator and the dot product calculator). However, fortunately, we'll limit ourselves to two basic ones which follow similar rules to the same matrix operations (vectors are, in fact, one-row matrices). First of all, we can add them:
Or:
And we can multiply them by a scalar (a real or complex number) to change their magnitude:
Or:
Truth be told, a vector space doesn't have to contain numbers. It can be a space of sequences, functions, or permutations. Even the scalars don't have to be numerical! But let's leave that abstract mumbo-jumbo to scientists. We're quite fine with just the numbers, aren't we?
Linear combination of vectors
Let's say that we're given a bunch of vectors (from the same space): , , , ..., . As we've seen in the above section, we can add them and multiply them by scalars. Any expression that is obtained this way is called a linear combination of the vectors. In other words, any vector , that can be written as
Where , , , ..., , are arbitrary real numbers is said to be a linear combination of the vectors , , , ..., . Note that is indeed a vector since it's a sum of vectors.
Okay, so why do all that? There are several things in life, like helium balloons and hammocks, that are fun to have but aren't all that useful on a daily basis. Is it the case here?
Let's consider the Cartesian plane, i.e., the 2-dimensional space of points with two coordinates, where and are arbitrary real numbers. We already know that such points are vectors, so why don't we take two very special ones: and . Now, observe that:
In other words, any point (vector) of our space is a linear combination of vectors and . These vectors then form a basis (and an orthonormal basis at that) of the space. And believe us, in applications and calculations, it's often easier to work with a basis you know rather than some random vectors you don't.
🙋 The vectors and are unit vectors. They have some special features we analyzed in detail at our unit vector calculator!
But what if we added another vector to the pile and wanted to describe linear combinations of the vectors , , and, say, ? We've seen that and proved enough to find all points. So adding shouldn't change anything, should it? Actually, it seems quite redundant. And that's exactly where linear dependence comes into play.
Linearly independent vectors
We say that , , , ..., are linearly independent vectors if the equation
(here is the vector with zeros in all coordinates) holds if and only if . Otherwise, we say that the vectors are linearly dependent.
The above definition can be understood as follows: the only linear combination of the vectors that gives the zero vector is trivial. For instance, recall the vectors from the above section: , , and then also take . Then
so we've found a non-trivial linear combination of the vectors that gives zero. Therefore, they are linearly dependent. Also, we can easily see that and themselves without the problematic are linearly independent vectors.
The span of vectors in linear algebra
The set of all elements that can be written as a linear combination of vectors , , , ..., is called the span of the vectors and is denoted . Coming back to the vectors from the above section, i.e., , , and , we see that
where is the set of points on the Cartesian plane, i.e., all possible pairs of real numbers. In essence, this means that the span of the vectors is the same for , , and , and for just and (or, to use formal terms, the two spaces' intersection is the whole ). This suggests that is redundant and doesn't change anything. Yes, you guessed it - that's precisely because of linear dependence.
The span in linear algebra describes the space where our vectors live. In particular, the smallest number of elements that is enough to do it is called the dimension of the vector space. In the above example, it was because we can't get fewer elements than and .
A keen eye will observe that, in fact, the dimension of the span of vectors is equal to the number of linearly independent vectors in the bunch. In the example above, it was pretty simple: the vectors and were the easiest possible (in fact, they even have their own name: the standard basis). But what if we have something different? How can we check linear dependence and describe the span of vectors in every case? In a minute, we'll find out just that and so much more!
How to check linear dependence
To check linear dependence, we'll translate our problem from the language of vectors into the language of matrices (arrays of numbers). For instance, say that we're given three vectors in a 2-dimensional space (with two coordinates): , , and . Now let's write their coordinates as one big matrix with each row (or column, it doesn't matter) corresponding to one of the vectors:
Then the rank of the matrix is equal to the maximal number of linearly independent vectors among , , and . In other words, their span in linear algebra is of dimension . In particular, they are linearly independent vectors if, and only if, the rank of is equal to the number of vectors.
So how do we find the rank? Arguably, the easiest method is Gaussian elimination (or its refinement, the Gauss-Jordan elimination). It is the same algorithm that is often used to calculate systems of equations, especially when trying to find the (reduced) row echelon form of the system.
🙋 If you want to learn how to calculate the rank of a matrix, visit Omni's matrix rank calculator for an in-depth analysis!
The Gaussian elimination relies on so-called elementary row operations:
- Exchange two rows of the matrix.
- Multiply a row by a non-zero constant.
- Add to a row a non-zero multiple of a different row.
The trick here is that although the operations change the matrix, they don't change its rank and, therefore, the dimension of the span of the vectors.
The algorithm tries to eliminate (i.e., make them ) as many entries of as possible. In the above case, provided that is non-zero, the first step of Gaussian elimination will transform the matrix into something in the form:
Where and are some real numbers. Then, as long as is not zero, the second step will give the matrix
Now we need to observe that the bottom row represents the zero vector (it has 's in every cell), which is linearly dependent with any vector. Therefore, the rank of our matrix will simply be the number of non-zero rows of the array we obtained, which in this case is .
That was quite enough time spent on theory, and we all know time is worth its weight in gold. Let's try out an example to see the linear independence calculator in action!
Example: using the linear independence calculator
Let's say that you've finally made your dreams come true - you bought a drone. You're finally able to take pictures and videos of the places you visit from far above. All you need to do is program its movements. The drone requires you to give it three vectors along which it'll be able to move.
The world we live in is 3-dimensional, so the vectors will have three coordinates. Not thinking too much, you take some random vectors that come to mind: , , and . But is it really worth it just closing your eyes, flipping a coin, and picking random numbers? After all, most of your savings went into the thing, so we'd better do it well.
Well, if you did choose the numbers randomly, you might find that the vectors you chose are linearly dependent, and the span of the vectors is, for instance, only 2-dimensional. This means that your drone wouldn't be able to move around however you wish, but be limited to moving along a plane. It might just happen that it would be able to move left and right, front and back, but not up and down. And how would we get those award-winning shots of the hike back if the drone can't even fly up?
It is fortunate then that we have the linear independence calculator! With it, we can quickly and effortlessly check whether our choice was a good one. So, let's go through how to use it.
We have vectors with coordinates each, so we start by telling the calculator that fact by choosing the appropriate options under "number of vectors" and "number of coordinates." This will show us a symbolic example of such vectors with the notation used in the linear independence calculator. For instance, the first vector is given by . Therefore, since in our case the first one was , we input
Similarly for the two other ones we get:
And:
Once we input the last number, the linear independence calculator will instantly tell us if we have linearly independent vectors or not, and what is the dimension of the span of the vectors. Nevertheless, let's grab a piece of paper and try to do it all independently by hand to see how the calculator arrived at its answer.
As mentioned in the above section, we'd like to calculate the rank of a matrix formed by our vectors. We'll construct the array of size 3×3 by writing the coordinates of consecutive vectors in consecutive rows. This way, we arrive at a matrix
We'll now use Gaussian elimination. First of all, we'd like to have zeros in the bottom two rows of the first column. To obtain them, we use elementary row operations and the from the top row. In other words, we add a suitable multiple of the first row to the other two so that their first entry will become zero. Since and , we add a multiple of and of the first row to the second and third row, respectively. This gives a matrix:
Next, we'd like to get in the bottom row in the middle column and use the to do it. Again, we add a suitable multiple of the second row to the third one. Since the multiple is . Therefore,
We've obtained zeros in the bottom rows. We know that the matrix's rank, and therefore linear dependence and the span in linear algebra, are determined by the number of non-zero rows. This means that in our case, we have , which is less than the number of vectors, and implies that they are linearly dependent and span a 2-dimensional space.
So the very thing that we feared might happen happened - our drone will have no freedom of movement. But we can't miss out on this chance to film all those aerial shots! Fortunately, we have the linear independence calculator at hand and can play around with the vectors to find a suitable vector combination. And once we have that, we pack up, get in the car, and go on an adventure!
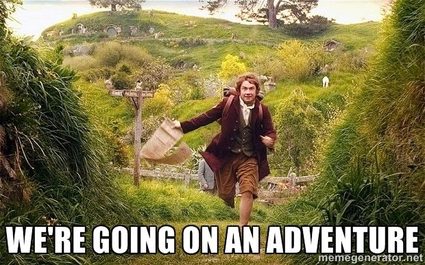
FAQ
How do I check if vectors are linearly independent?
You can verify if a set of vectors is linearly independent by computing the determinant of a matrix whose columns are the vectors you want to check. They are linearly independent if, and only if, this determinant is not equal to zero.
Are [1,1] and [1,-1] linearly independent in R²?
Yes, vectors of [1,1] and [1,-1] are linearly independent. We can see that by computing the determinant: 1 × (-1) - 1 × 1 = -2
.
Do 2 arbitrary vectors span R²?
Two arbitrary vectors do not necessarily span R². Two vectors will span R² if, and only if, they are linearly independent. An example of two vectors that span R² are [1,1]
and [1,-1]
. An example of two vectors that do not span R² are [1,1]
and [3,3]
.
Can 2 vectors span R³?
No, two vectors are not enough to span R³. You need at least three vectors to span R³. A given set of three vectors will span R³ if, and only if, they are linearly independent.
Is the identity matrix linearly independent?
Yes, the columns of the identity matrix form a linearly independent set: the determinant of the identity is equal to one.
|